Manual Review is the process of human reviewers painstakingly reviewing documents collected as part...
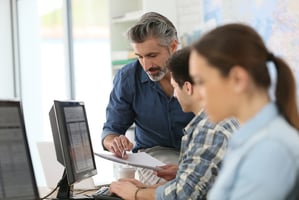
Active learning is the AI within RelativityOne’s analytics suite.
We have recently completed a large-scale review for an investigation. Time and cost were, as they always are, huge issues, so this was a fantastic project to show off the capabilities and spectacular accuracy of active learning.
The case initially had over 1.5 million documents. After filtering, keyword and concept searching, about 600,000 documents were left to review. When you start a case, visualise that you have a huge mountain of documents to look at.
With active learning running in the background, the system watches and analyses the decisions that you make, right from the very first document that is reviewed and tagged relevant or not relevant.
All the while, active learning is still working in the background, analysing your decisions and improving its own accuracy.
In a typical review, the two document mountains will be fairly close together but, as the review goes on, you want them to move further and further apart. You want the valley between the documents to become more and more sparse, as these are the documents that the system is not sure about.
As your mountains separate, so does the risk of relevant or hot documents remaining unreviewed and lost within the not-relevant documents.
You carry on with prioritised review until the flow of relevant documents that the system is serving you dries up. Then you would move to a coverage review.
A coverage review is a review of those documents in the ‘valley’ that the system is unsure about. The system analyses the decisions you make on these documents and subsequently learns and improves its own accuracy, further separating those mountains and reducing the documents between them.
Now is probably a good time to run what we call an elusion test. What’s an elusion test?
This is where we test the system to see if the documents marked not relevant, and are therefore being discarded, might actually contain relevant documents. Delve into a more in-depth look into the Elusion Test.
The system starts serving the reviewers' documents that it feels are not relevant. If we find a relevant document, the test is considered a fail and we go back to a prioritised review.
During elusion testing, using the magic of statistical analysis, we measure how well the system is performing, and ask ourselves, 'if we stop reviewing now, how many documents could we potentially miss?' Of course, this becomes a matter of proportionality.
You would continue until you feel that the chance of finding any further relevant documents would be disproportionate to the resources and cost required to find them.
In the review that we mentioned earlier, we initially had over half-a-million documents. Following filtering and searches, however, less than 20 per cent — about 90,000 — of those documents were actually reviewed, as our elusion tests were showing just a 0.59 per cent chance that we might find anything else relevant.
This showed a 99.44% accuracy.
Stay up to date with all things eDiscovery and information governance. Weekly updates ensure you’re always in the loop.
Manual Review is the process of human reviewers painstakingly reviewing documents collected as part...
Ever since Relativity announced their new GenAI aiR suite of tools based on OpenAI's GPT-4 model,...
Since the launch of Chat GPT in November 2022, there has been an unprecedented uptake in the use of...